Innovating Agriculture: Palm Oil Fruit Grader
- danielle phua
- Feb 26, 2020
- 3 min read
Find out how Vision AI, remote sensing and Q-GIS technologies were used to revolutionise the grading process, enhancing both accuracy and efficiency in the palm oil industry.
The palm oil industry is a critical economic driver in many parts of Southeast Asia, but it faces significant challenges in maintaining quality and efficiency. Traditional methods of grading palm oil often involve manual inspection, which can be time-consuming and prone to human error.
Recognizing the need for innovation, I led the design of a Vision AI-powered solution to automate and enhance the grading process, ensuring higher accuracy and efficiency.
Challenge
Inconsistent grading of palm oil bunches was leading to significant losses for both producers and buyers. The manual inspection process was not only labor-intensive but also subjective, leading to discrepancies in quality assessment.
The industry needed a more reliable, scalable solution to ensure consistency in quality while reducing the reliance on manual labor.
Approach
Research & Field Study:
Conducted extensive onsite research, including shadowing local graders and engaging with industry stakeholders to understand the nuances of the grading process and the specific challenges faced.
AI Integration:
Collaborated with a multidisciplinary team to integrate Vision AI technology into the grading process. The AI system was trained to identify key quality indicators, such as ripeness and defect levels, ensuring a consistent and objective grading process.
User-Centered Design:
Focused on creating an intuitive interface for the graders, allowing them to easily interact with the AI system. The design emphasized simplicity and clarity, ensuring that even those with minimal technical knowledge could effectively use the tool.
Pilot Testing & Iteration:
Deployed the system in a pilot program, gathering feedback from users and continuously iterating on the design to improve accuracy and user experience.
AI Constraints
Laid out, top down Taken from far Overlapping fruits
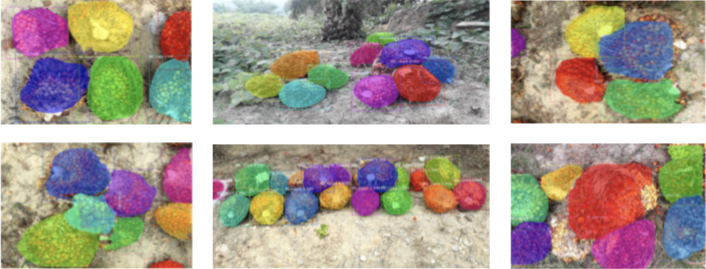
Best Performance of Counting AI Worst
<-------------------------------------------------------------------------------------------------------------------------
Slowest Speed of count & grade Fastest
Outcome:
Increased Grading Accuracy:
The Vision AI system achieved a grading accuracy of over 95%, significantly reducing the margin of error compared to manual methods.
Operational Efficiency:
The automated process cut grading time by 50%, allowing for quicker processing and reducing the overall cost of labor.
Scalability:
The solution was scalable, with the potential to be deployed across multiple regions, ensuring consistency in grading standards across the industry.
Research Methodologies
Onsite Observations and Shadowing:
Conducted field visits to palm oil plantations and processing facilities to observe the existing grading process. Shadowed local graders to gain a first-hand understanding of the challenges and nuances involved in manual grading.
Stakeholder Interviews:
Engaged with key stakeholders, including plantation owners, graders, and buyers, to gather insights into their needs, pain points, and expectations from an automated grading system.
Technology Feasibility Study:
Collaborated with technical experts to assess the feasibility of integrating Vision AI technology into the grading process. This involved testing various AI models to determine their effectiveness in identifying key quality indicators in palm oil bunches.
Contextual Inquiry:
Performed contextual inquiry by immersing myself in the environment where grading takes place, allowing for a deeper understanding of the conditions and factors influencing grading accuracy and efficiency.
Iterative Prototyping and Testing:
Developed and tested prototypes of the Vision AI system in a controlled environment before deploying them in the field. Collected feedback from users during pilot testing to refine the system and improve its usability and accuracy.
Key Learnings
Human-AI Collaboration:
The importance of designing systems that complement and enhance human capabilities, rather than replace them.
Iterative Design:
The value of continuous feedback and iteration in refining the AI system to meet real-world needs effectively.
Contextual Understanding:
The need to deeply understand the specific challenges and context of the industry to design solutions that are both practical and impactful.
Comentarios